ORI Awards Three Research Integrity Grants
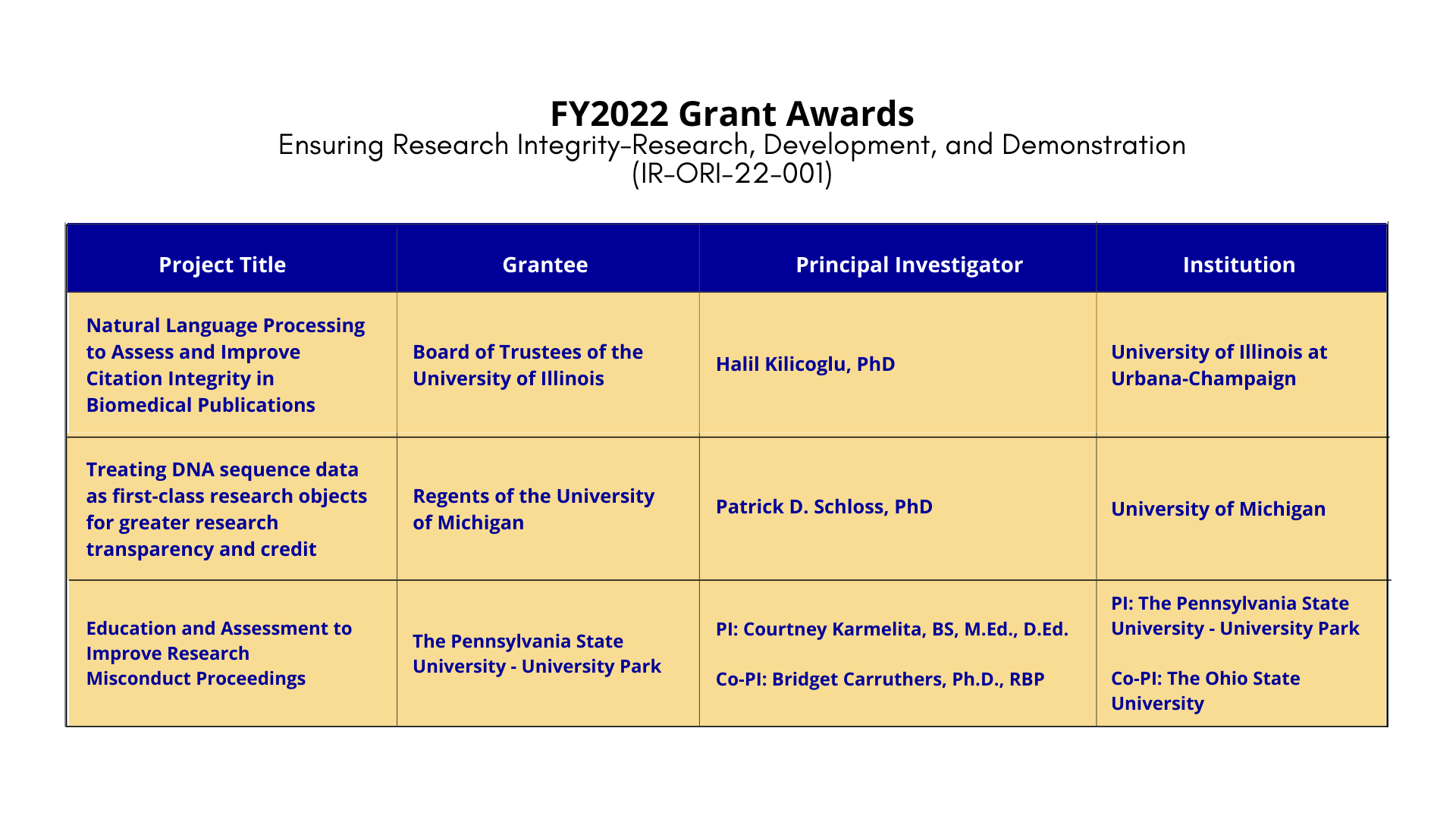
Project Title: Natural Language Processing to Assess and Improve Citation Integrity in Biomedical Publications
Grantee: Board of Trustees of the University of Illinois
Principal Investigator: Halil Kilicoglu, PhD
Institution: University of Illinois at Urbana-Champaign
Abstract:
Trustworthy science is crucial to scientific progress, evidence-based policies, and human health. Problems in research design, conduct, and reporting threaten scientific integrity, waste resources, and risk a loss of public trust in science. Citations play a fundamental role in diffusion of scientific knowledge[1] and research assessment[2]; yet their role in research integrity is often overlooked[3]. Citation inaccuracies (e.g., citation of non-existent findings[3]) undermine the integrity of the biomedical literature, distorting the perception of available evidence[4] with potentially serious consequences for human health. A recent meta-analysis showed that 25.4% of medical articles contained a citation error[5]. Retracted articles continue to be cited positively years after being retracted, spreading scientific misinformation[6,7]. A bibliometric analysis revealed that inaccurate citations of a letter published in 1980[8] may have contributed to the opioid crisis[9]. Considering such negative consequences, it is critical to ensure that the scientific contents of all referenced articles are accurately and properly cited in a manuscript before its publication. However, assessing citation accuracy requires considerable manual effort. Authors often take shortcuts, copying citations from other articles without checking their accuracy[10]. Journals and peer reviewers lack the resources to verify manuscripts for citation inaccuracies. Automated tools that can identify citation inaccuracies would help authors, journals, and peer reviewers in mitigating the negative consequences of citation distortions and improve transparency and integrity of scholarly communication[11]. The objective of this project is to develop scalable natural language processing (NLP) and artificial intelligence (AI) algorithms to automatically assess biomedical publications for citation content accuracy. The resulting models can be embedded in practical software tools. With these new tools, authors will be able to improve their citation quality; journals and peer reviewers will be able to scrutinize questionable citation practices pre-publication; and research administrators, research integrity officers, funders, and policymakers will be able to investigate citation practices, integrity issues, and knowledge diffusion via citations.
Toward this objective, in this first year of the project, we will construct a corpus of 100 highly cited articles based on PHS-funded research and 20 articles citing each of these articles, align the citation context in the citing article with the relevant text spans in the reference article, and assess whether the citation is accurate with respect to the reference article.
In the second year, we will use the resulting corpus to train and validate AI-based NLP models that identify related text spans in reference-citing article pairs and calculate a confidence score for citation accuracy. The proposed work is innovative because: (a) it is the first project focusing on automated citation accuracy verification in biomedical publications; (b) it tackles the significant NLP challenge of aligning the content of two articles at multiple levels of granularity (e.g., single sentence, passage, entire article). To address these challenges, we will leverage state-of-the-art sentence encoders, such as BERT[12], as well as long document encoders, such as Longformer[13], and a multilevel text alignment approach[14].
The models developed will serve various stakeholders in improving citation quality and ensuring citation transparency and integrity. In addition, the proposed corpus and models will stimulate research in citation content analysis[15], contributing to development of more granular and accurate measures of scholarly impact. In the longer term, such qualitative measures can mitigate the detrimental effects that purely quantitative metrics of research assessment have had on research integrity and quality[16].
1. Greenberg SA. Understanding belief using citation networks. Journal of evaluation in clinical practice. 2011;17(2):389–393.
2. Waltman L. A review of the literature on citation impact indicators. Journal of Informetrics. 2016;10(2):365– 391.
3. Pavlovic V, Weissgerber T, Stanisavljevic D, Pekmezovic T, Milicevic O, Lazovic JM, et al. How accurate are citations of frequently cited papers in biomedical literature? Clinical Science. 2021;135(5):671–681.
4. Jannot AS, Agoritsas T, Gayet-Ageron A, Perneger TV. Citation bias favoring statistically significant studies was present in medical research. Journal of clinical epidemiology. 2013;66(3):296–301.
5. Jergas H, Baethge C. Quotation accuracy in medical journal articles—a systematic review and meta- analysis. PeerJ. 2015;3:e1364.
6. Van Der Vet PE, Nijveen H. Propagation of errors in citation networks: a study involving the entire citation network of a widely cited paper published in, and later retracted from, the journal Nature. Research integrity and peer review. 2016;1(1):1–10.
7. Schneider J, Ye D, Hill AM, Whitehorn AS. Continued post-retraction citation of a fraudulent clinical trial report, 11 years after it was retracted for falsifying data. Scientometrics. 2020;125(3):2877–2913.
8. Porter J, Jick H. Addiction rare in patients treated with narcotics. The New England journal of medicine. 1980;302(2):123–123.
9. Leung PT, Macdonald EM, Stanbrook MB, Dhalla IA, Juurlink DN. A 1980 letter on the risk of opioid addiction. The New England journal of medicine. 2017;376(22):2194–2195.
10. Gavras H. Inappropriate Attribution: The “Lazy Author Syndrome”. American Journal of Hypertension. 2002;15(9):831–831.
11. Kilicoglu H. Biomedical text mining for research rigor and integrity: tasks, challenges, directions. Briefings in bioinformatics. 2018;19(6):1400–1414.
12. Devlin J, Chang MW, Lee K, Toutanova K. BERT: Pre-training of Deep Bidirectional Transformers for Language Understanding. In: Proceedings of the 2019 Conference of the North American Chapter of the Association for Computational Linguistics: Human Language Technologies, Volume 1 (Long and Short Papers). Minneapolis, Minnesota: Association for Computational Linguistics; 2019. p. 4171–4186.
13. Beltagy I, Peters ME, Cohan A. Longformer: The long-document transformer. arXiv preprint arXiv:200405150. 2020.
14. Zhou X, Pappas N, Smith NA. Multilevel Text Alignment with Cross-Document Attention. In: Proceedings of the 2020 Conference on Empirical Methods in Natural Language Processing (EMNLP). Online: Association for Computational Linguistics; 2020. p. 5012–5025.
15. Zhang G, Ding Y, Milojević S. Citation content analysis (CCA): A framework for syntactic and semantic analysis of citation content. Journal of the American Society for Information Science and Technology. 2013;64(7):1490–1503.
16. Hicks D, Wouters P, Waltman L, De Rijcke S, Rafols I. Bibliometrics: the Leiden Manifesto for research metrics. Nature. 2015;520(7548):429–431.
Project Title: Treating DNA sequence data as first-class research objects for greater research transparency and credit
Grantee: Regents of the University of Michigan
Principal Investigator: Patrick D. Schloss, PhD
Institution: University of Michigan
Abstract:
Starting in January 2023, the NIH is requiring that researchers release the published and unpublished data related to their funded projects. Less than a year away, many researchers seem unaware of the policy change. Yet, making data publicly available is critical to transparency in the reporting of results and the ability of others to reproduce and build upon those results. The community of researchers that generate DNA sequence data have a culture of making their data publicly available prior to publication and present a unique opportunity to evaluate open data practices before and after implementation of the NIH policy. At the same time, many publishers are strengthening their open data policies and encouraging researchers to treat their data as “first-class research objects” that need to be cited to provide proper attribution and access to the data underlying a study. The overall objective of the proposed research is to create a case for researchers to engage in open data practices. Our rationale is that if scientists can see the benefits of engaging in open practices, NIH-funded researchers and others will be more likely to adopt them. The approach we will take is based on our long standing work in disseminating reproducible research practices and our leadership within the American Society for Microbiology (ASM) Journals program. Our proposed research has two specific aims that include: (1) quantifying the adherence to data accessibility policies for sequencing data at microbiology journals and (2) assessing the adoption and impact of implementing data citation standards at a large microbiology research publisher. At the end of the proposed project, we expect to have quantified the benefits of using open data practices, which we will use to encourage scientists to make their data open and citable. The parallel implementation of the NIH Policy for Data Management and Sharing and ASM’s adoption of data citations makes this an ideal time and venue for the proposed effort.
Project Title: Education and Assessment to Improve Research Misconduct Proceedings
Grantee: The Pennsylvania State University - University Park
Principal Investigator: Courtney Karmelita, BS, M.Ed., D.Ed.
Institution (PI): The Pennsylvania State University - University Park
Co-Principal Investigator: Bridget Carruthers, Ph.D., RBP
Institution (Co-PI): The Ohio State University
Abstract:
The focus area of this proposal is “Handling allegations of research misconduct under 42 C.F.R. Part 93”. the proposed research will address the need for education and resource development for individuals assisting with the handling of research misconduct allegations at the inquiry or investigation phases. To date, there has been no published research on this topic as most Responsible Conduct of Research (RCR) training emphasizes the prevention of research misconduct rather than the policy and processes that govern the handling of research misconduct allegations. The study team will conduct a needs assessment through benchmarking with other institutions to identify and prioritize the creation of educational materials and trainings. The study team will then use the needs assessment to create online modules to provide foundational knowledge of research misconduct processes, definitions, and procedures for inquiry officials and investigation committee members. The study team will then implement the newly created resources and seek feedback from the appropriate stakeholders. In addition, the study team will explore to what extent, if any, standing committees develop a deeper understanding of the research misconduct process. This will help to inform which, if either, committee type is more efficacious. Research on standing versus ad hoc committees is also lacking in the literature. This proposed research has the potential to positively impact the research integrity community at large with much needed tools, resources, training and research on the management of research misconduct allegations.